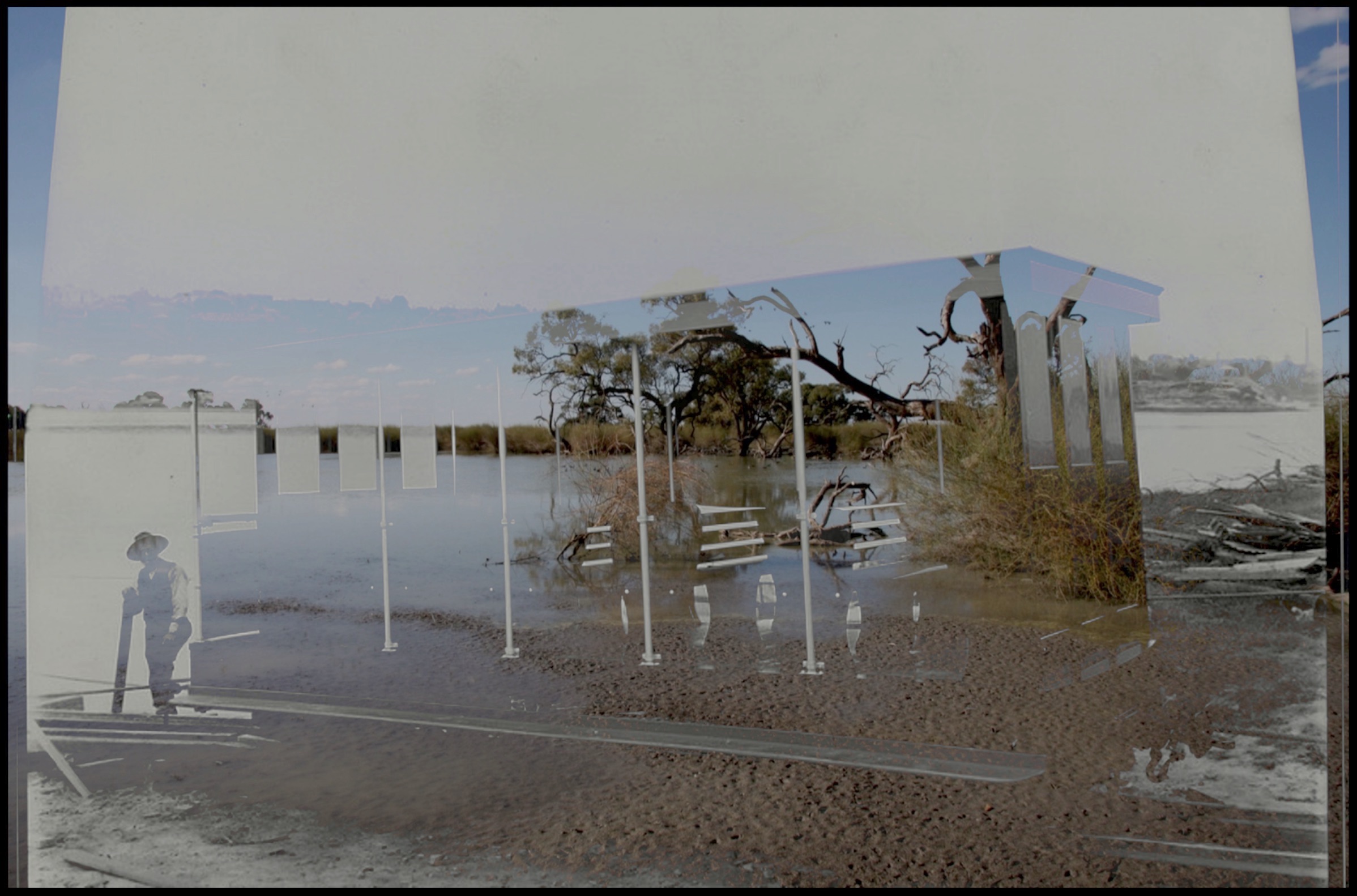
This article discusses two practical experiments in remaking collections. Drifter (2016) and Succession (2014) build on the affordances of machine-readable collections and APIs to harvest large datasets from diverse sources, and show how these sources can be re-deployed to address complex spatiotemporal sites. These projects demonstrate the potential of a mashup-like generative approach based on sampling and recombination. Such approaches generate an expansive range of unforeseeable outcomes, while retaining a highly authored character. Here these projects are analysed through three key constituents: the troublesome trace of data; their extraction of digital samples; and their generative recomposition of samples into emergent outcomes. These techniques remake collections in a way that addresses the intrinsically complex, entangled and heterogeneous nature of what Latour terms ‘matters of concern’.